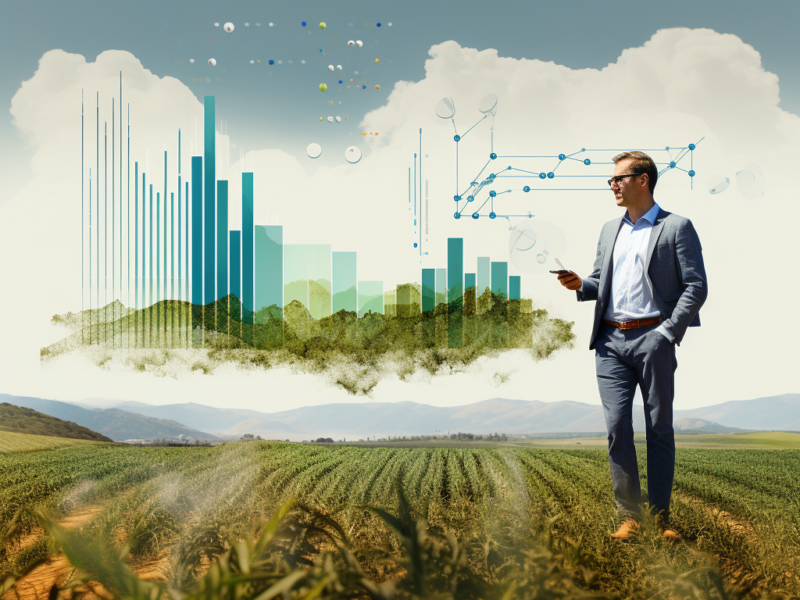
Cultivating Ag IQ: Using Data to Originate More Ag Loans
Want this content in a PDF? Download it here
For most lenders finding data is not a problem, in fact access to too much data is normally the problem. How data is structured, stored and managed can become an asset or a liability for a lender with regard to how they do business, or with regard to mergers and acquisitions, as outlined in this article: I don’t want your data problem.
Not storing, managing, or organizing data can lead to enormous tech debt and poor, inefficient processes, and ultimately become a major liability for any company. However, if used properly data can be an asset that helps banks better serve their customers and a competitive advantage that helps them win more business.
For the purposes of this article we will focus on one specific data set: data that can help drive more loans. This can be internal customer data, or other data the bank possesses, as well as licensed third party data sets that can help an Ag lender not only identify new clients, but understand key information about new and existing clients that will help them close more business.
Show me the data
To start, let’s look at the data sets available to lenders that can help them close more business:
- Existing mortgage data
- Operator data
- UCC Filings
- Ownership data
- Transaction data
- Existing customer data
The first two data sets are readily available.
Mortgage data and UCC filing data is publicly available and there are several companies that license the data in an aggregated and normalized format for private use. The original consumers of these data sets were commercial and residential real estate lenders and equipment and automotive finance companies. Although extremely relevant to agriculture, the data sets weren’t designed specifically for use by agriculture lenders and thus lack important context.
The third data set, operator data, is specific to agriculture and can be licensed from a small set of data aggregators. This data can help you understand operator personas, provide contact information, as well as provide insight into their operation, i.e.: how big are they, where and what do they farm?
Transaction data and ownership data is also readily available through several data aggregators, however, it can be challenging to make efficient use of these data sets.
Transactions specifically are messy and require a lot of sanitization to identify true arms lengths transactions, a problem that entire appraisal teams dedicate themselves to solving.
The last data set is the most important, your client data. Who is a customer, or a prospect, who owns the relationship, and how do you do business with them today? This information is usually in your CRM like Salesforce.
Using the Data
Agriculture lenders are already using these data sets to close more loans. They ingest mortgage and UCC filing data to identify the biggest buyers of land in their area, to identify prospective clients in their territory, to develop marketing lists that target competitive banks and to understand
their own market position. Combined with knowledge of competitors’ products and when loans are originated they develop tactics to reach out at times when they know a customer will likely be open to re-financing.
Marketing teams license and use the operator data to build campaigns. Loan officers have access to the lists to create their own call lists, or research specific customers. Some data providers will also offer platforms where additional context is provided and tools for a loan officer
or marketing professional to perform additional research and/or create specific lists.
Ownership data, like operator data can be downloaded in lists and mined for information. Several platforms exist for loan officers to login and do their own research on a client to understand how much land they own. Many also will provide additional information on the estimated value of a
property. However, the transaction data available in those platforms in most cases is actually inferior to the transaction data that a lender already has access to through their appraisal groups, but in most cases this data is not readily available, or usable by loan officers or marketing groups,
as the intra-company data connections to surface that data with the ag appropriate context have not been made.
The Data Problem for Loan Officers
The above data sets are useful and they can help a loan officer close more loans, which is why many Ag lenders license at least one of the data sets listed above and employ some or all of the tactics outlined above to identify new clients. The challenge is the time, effort and work to make
the data actionable. This normally falls to a group within an Ag lender that crunches the numbers, or perhaps tries to combine data sets to add additional context. However, even then, the output is tabular data with limited visuals that is hard for most loan officers to use effectively, or access.
A loan officer may also try to gather the data directly using the tools provided by the data provider, however, this is also challenging because the data resides in different platforms. A loan officer might have to manage several logins and go to several sites to find the data they want or need.
This could be the data providers app, as well as an internal intranet where the data team hosts a power BI report, or where the appraisal group publishes sales trends. To further compound the problem, the data is rarely matched with current clients and prospects.
We have heard from customers that great loan officers will take the time to sift through the data to build a client profile, but that task can take hours, which means less time selling for the loan officer.
The Perfect Solution with Ag Context
The first part of a perfect solution is to add a dash of agricultural context. Plat Maps, bushels (lbs, bales, etc.), and acres – this is the language of farmers and the language of agriculture. The first step to solving the problem is ensuring that the data is not only tabular, but can be viewed in the
context of Agriculture: plat maps, bushels and acres. Every agriculture professional can instantly form an opinion about a property if they see a satellite image and have access to a map. Add in a soil map, crop history, topographic data and most people will form a strong opinion. However, this
also applies to looking at a farmer’s operational footprint, the parcels a mortgage is tied to, the land they own, the equipment they are buying and more more. With the right context, inferences not only come quicker, but they tend to be more accurate.
Consider the following data and the context, how would you prefer to consume the data?
Transactional Data
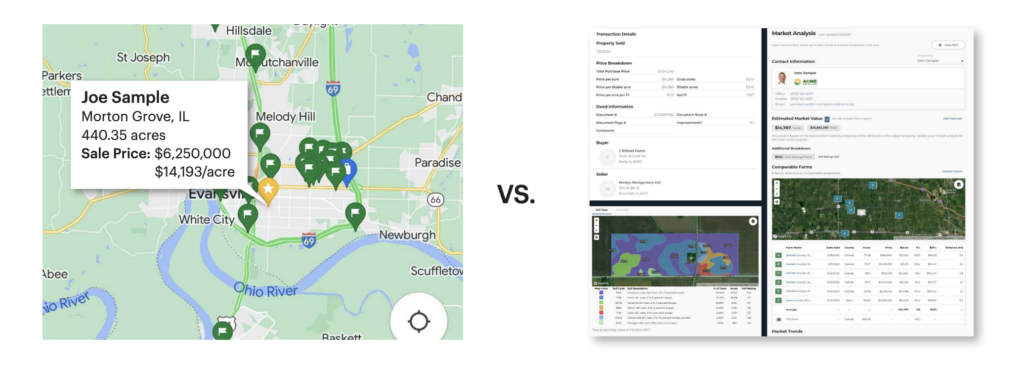
Mortgage and UCC Filing Data
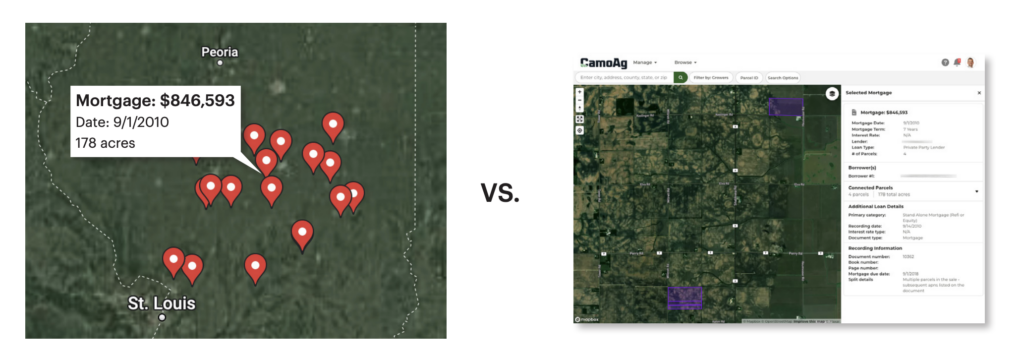
Operational Footprint
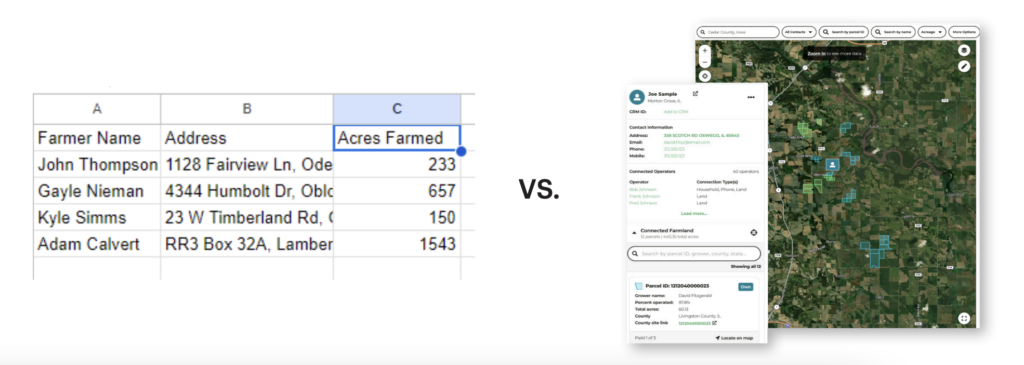
The second part of a perfect solution is viewing all of the data together, including matching licensed data sets to your current clients and prospects. Not only is going to multiple sites to gather and match data on a current client or prospect frustrating, it is not an efficient use of time. It is also more than likely that important connections in the data are being missed. In fact, when data is combined, it becomes more powerful. A large operator that is heavily mortgaged, is not the same as a large operator that owns all of their land free and clear.
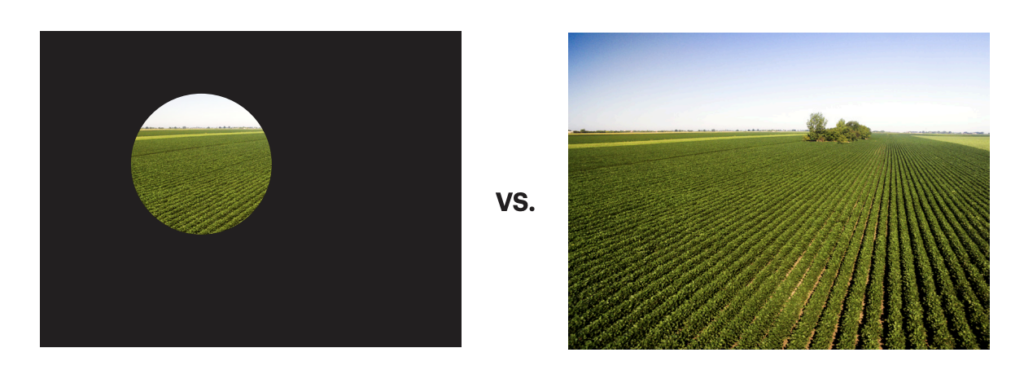
Approaches to Creating the Perfect Data Solution for Ag Lending
Creating the perfect solution is not easy. Here are a couple of approaches we have seen and the benefits and pitfalls of those approaches.
Depend on a data provider.
Today you may use a certain data provider and they may have a good platform and it may seem logical to ask them to help connect the data and provide the much needed context. The fact that they invested in a platform proves the point that data without context is less valuable, otherwise data providers would just send spreadsheets and spreadsheets worth of data to their customers instead of developing tools to provide context and help them better sort through the data. The benefit of this approach is it is cheapest from an op-ex perspective, however, there are several problems with depending exclusively on a data provider to help build the perfect solution:
- A data provider’s tools can’t make the last and most important data connection – the connection back to your customers. And giving your customer data to a company whose business is selling data, just isn’t good business
- It is nearly impossible to get all of the data from one company. Even the biggest and best lack a couple key ingredients and normally data providers don’t like giving their data to other data companies. It’s also rare that a data provider has the best data across all categories, each has their strengths and weaknesses
- Lastly, there is the risk that the data provider’s data goes bad – what happens if the data provider you choose is no longer able to provide the best data in a category, or another data set becomes available that they don’t have? The investments in connecting the data, training the team, and building new processes are all lost.
Build it yourself.
To overcome the challenges and risks of depending on a data provider to help solve the problem and build the perfect solution, some ag lenders with the budget and access to IT resources might consider building the solution themselves. There are several down sides
to this approach:
- The initial development cost
- Ongoing support costs
- And the time it takes to develop the solution.
The benefit of this approach is complete control. The cost of this approach also likely causes an organization to skip an important step in
building a solution that includes agricultural context. This is because doing so requires major investments in a geospatial platform and foundational data sets that aren’t core to the objective, but remain very important to adding context and utility to the final product. For example, consider the need to map all tax PINs and parcels, CLUs, SSURGO soil data, USDA data and more – this data is secondary to solving the core problem and it is tempting to leave it out as the investment in the technology to include it is substantial. However, as outlined above, without this context, the data is simply visualized as “pins” on a map, which leaves out important context and fails to accurately visualize the underlying connections buried within the data.
Based on conversations with ag lenders that have considered this approach and our own estimates, it would conservatively cost $2M-$5M in upfront investment and 18-24 months of development time to build the solution in house. The ongoing costs of supporting such a solution, including data hosting, and third party software licenses (i.e.: ESRI) range from $1M to $1.5M annually depending on the complexity of the solution and the cost efficiency of the IT department.
License a purpose built solution for ag lenders.
Up until now, this has not been an option available to ag lenders. However, CamoAg has taken this approach and is offering a purpose built solution to
ag lenders designed to address the pitfalls of alternatives.
A Farm Credit Association approached CamoAg to help develop a solution that can provide agricultural context and match disparate data, including customer data through an integration with Salesforce / nCino. The benefit of this approach is the proven advantages of a Software as a Service business model, mainly that the costs are lowered and that the solution improves through the shared investment of all customers. The one downside is that users do give up some control over the actual implementation, however, this is a challenge that is regularly overcome by SaaS providers by involving customers in the development and prioritization of improvements and new capabilities for a product. With this association as our first customer, we developed and deployed a solution in 6 months at a fraction of the cost it would have taken them to develop it themselves.
Connecting Data vs. Selling Data
As part of our engagement with our first customer, CamoAg made a deliberate decision to focus on connecting data and not selling data to avoid conflicts of interest. We designed the solution to use the smallest cross section of non-confidential customer data possible to accurately
match customers to other data sets and through an integration with Salesforce, confidential and sensitive customer data remains in Salesforce. This decision, as well as an architecture that makes the solution data agnostic, enables the CamoAg Client Intelligence solution to integrate
with the preferred data providers of our customers, this decision also means that CamoAg is not in competition with data providers, but instead a partner – by adding additional context to their data and helping to connect it to other data, we make all data more valuable, helping data providers also grow their businesses.